Artificial Intelligence applied to medicine. In search of healthy ageing.
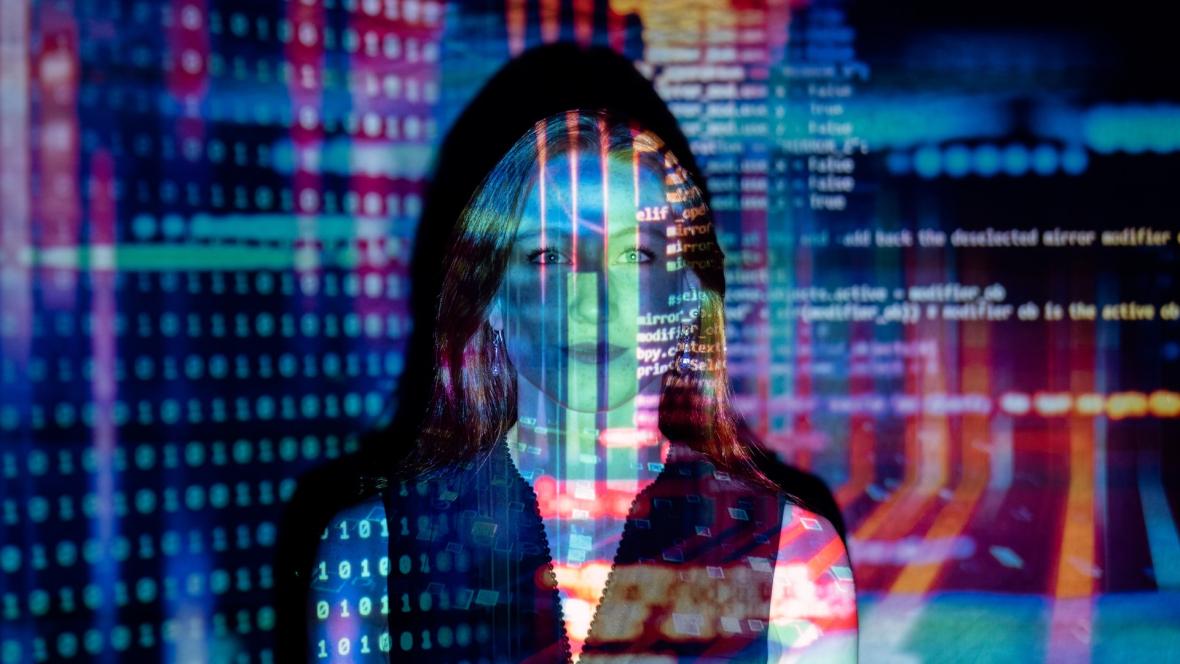
How to apply Artificial Intelligence in the quest for longevity
We age by nature and when the time comes, the most important thing is to do so in the best possible way. This affects the population as a whole, and science is working hard to try to find those advances that will bring us one step closer to the solution.
Specifically, research into conditions such as cancer, cardiovascular or neurodegenerative diseases is an area of medicine that is experiencing a boom in research and the application of technologies based on Deep Learning and Machine Learning.
Random forest classification for predicting lifespan-extending chemical compounds 1, is the name of the paper on which this post focuses and which explains how machine learning algorithms have been implemented on an extensive database of drugs to predict which chemical compounds extend the lifespan of Caenorhabditis elegans.
Caenorhabditis elegans
Caenorhabditis elegans is a species of nematode that has been widely used since the 1970s for various genetic studies, especially in developmental genetics. This is due to a number of characteristics that favour its study:
- It is transparent at all stages of its life, which makes it easy to observe.
- It is a hermaphrodite species, making it easier to obtain and maintain individuals.
- Its genetic simplicity, with only 959 cells, and a highly defined digestive and nervous system.
- In the laboratory, it is easy to maintain and feed.
- Its life cycle is relatively short (two to three weeks) making it possible to obtain results of studies applied at any time of its existence in a rapid manner.
- It is an organism in which the function of specific genes can be disrupted with relative ease, making it possible to silence specific functions and infer their effect.
Displacement of a C. elegans.
Several previous studies on ageing have shown that certain dietary restrictions, genetic modifications and drug interventions can extend the lifespan of model organisms. After compiling the findings from these studies and building on the work of Barardo 2 where he built a Random Forest model to predict whether a compound would increase the lifespan of C. elegans, Kapsiani and Howlin have further studied data from the DrugAge database.
In our study, five Random Forest predictive models were constructed, each with different types of descriptors: molecular fingerprints and/or pre-computed molecular descriptors.
The novelty of this work at the medical level is precisely the implementation of molecular fingerprints to build machine learning models. As for the more technological part of the research, Random Forest algorithms have been applied.
Random Forest Algorithms
Random Forest algorithms are one of the supervised learning techniques. Its basis is a combination of predictor trees in such a way that none of the trees by itself sees all the training data, so that each of these trees is trained with different data samples for the same problem.
With this technique of individual training and combining their total results, errors in the predictions that may arise for one of the trees are compensated by the results of the others and we have a prediction that works best for an overall problem.
Operation flow of a Random Forest.Analytics Vidhya
Conclusions
The main conclusion of this interesting study, beyond the technical results that can be explored in its reading, is that the application of artificial intelligence to studies where the number of variables is very high and a large amount of data is moved offers good results if applied correctly.
Medicine, as the main reference in studies of this type, is making constant and increasing use of these techniques. As this research shows, the results are getting better and better, which means that medical advances are becoming faster and faster, having a direct impact on society, health, quality of life and therefore on our longevity.
References
-
Kapsiani, S., Howlin, B.J. Random forest classification for predicting lifespan-extending chemical compounds. Sci Rep 11, 13812 (2021) Article Google Scholar
-
Barardo, D. et al. The DrugAge database of aging-related drugs. Aging Cell 16, 594–597 (2017). CAS PubMed PubMed Central Article Google Scholar